Beyond the Algorithm: A Leader's Strategic Framework for Successful Healthcare AI Adoption
- Dr Ajay Bakshi
- Apr 16
- 6 min read
Updated: Apr 28
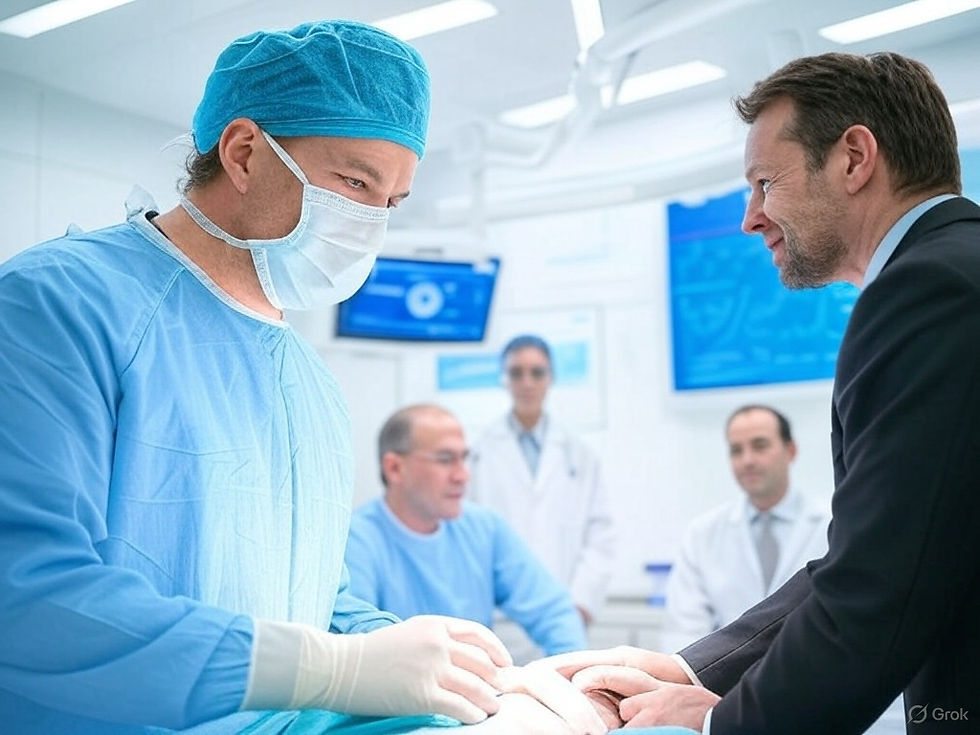
In our last discussion, we performed a reality check on the AI healthcare revolution, acknowledging both its immense potential and the significant hurdles from the perspectives of the clinic, the corner office, and the code. Now, we move beyond acknowledging the landscape to navigating it effectively. The critical question shifts from 'What can AI do?' to 'How can we, as leaders, strategically adopt AI to genuinely improve healthcare?'
Simply acquiring cutting-edge algorithms isn't enough. Successful, sustainable AI adoption in the complex, high-stakes environment of healthcare demands a deliberate, holistic strategy. It requires looking far beyond the technology itself. Over my years leading hospital systems and advising on healthcare transformation, I've seen that success hinges on building a robust foundation across several key domains.
Here is a strategic framework for successful healthcare AI – six essential pillars – that healthcare leaders should consider when charting their course for AI adoption:
1. Vision & Strategy: Anchoring AI to Purpose
Before a single line of code is written or procured, ask "Why?" What specific, critical organizational goals will AI help achieve? Are we aiming to demonstrably improve patient outcomes for a specific condition, significantly enhance operational efficiency in a bottlenecked area, or expand access to care for underserved populations?
The Work: Define clear, measurable objectives for AI initiatives. Ensure tight alignment with your organization's core mission and strategic priorities. Resist the allure of "AI for AI's sake." This internal clarity is more crucial than ever. Leaders today are understandably inundated with proposals from a rapidly growing number of AI innovators, each promising transformative results. It can be tempting to engage with the most persistent, articulate, or technologically intriguing proposals as they arise. However, reacting opportunistically without a clear internal strategy – this foundational 'Why?' – risks fragmentation. You might end up with a collection of exciting pilot projects that consume significant resources and bandwidth but don't integrate well, don't scale, and ultimately don't move the needle on your core organizational goals. This framework, particularly starting with Vision & Strategy, provides the necessary filter. It helps leaders proactively define their priorities first, allowing them to evaluate external proposals based on strategic fit, rather than being driven solely by external pitches. Prioritize initiatives based on genuine clinical need and potential impact identified internally.
Leadership Lens: From the CEO's chair, initiatives without clear strategic linkage often flounder, failing to secure sustained resources or buy-in – this is doubly true when those initiatives originate reactively from external pitches rather than internal strategy. From the clinic floor, tools disconnected from real workflow problems or patient needs are quickly ignored, regardless of how innovative the underlying tech might seem. Your AI vision must resonate across the organization, from the boardroom to the bedside, and serve as the primary guide for evaluating all potential AI endeavors, internal or external.
2. Data Readiness: Fueling AI Responsibly
AI algorithms are voracious consumers of data; it's their lifeblood. The quality, structure, and accessibility of your data will fundamentally determine the success and safety of any AI application.
The Work: Conduct a thorough assessment of your data assets – quality, completeness, potential biases, and accessibility. Establish robust data governance policies covering ownership, usage, quality standards, and lifecycle management. Implement stringent data privacy and security measures that comply with (and often exceed) regulations like HIPAA or GDPR – patient trust is paramount. Invest in the necessary infrastructure for data storage, processing, integration, and security. Break down detrimental data silos.
Leadership Lens: My background in science underscores the principle of "garbage in, garbage out." As a hospital leader, I grappled with fragmented legacy systems. In healthcare AI, where decisions impact lives, the standard for data integrity, security, and ethical sourcing must be exceptionally high. This isn't just an IT task; it's a core strategic enabler requiring executive oversight.
3. Ethical & Governance Guardrails: Building and Maintaining Trust
The power of AI brings significant ethical responsibilities. Proactively establishing clear ethical guidelines and governance structures is crucial for building trust with clinicians, patients, and regulators, and for ensuring equitable outcomes.
The Work: Develop and institutionalize clear ethical principles for AI development and deployment within your organization. Create multi-disciplinary oversight committees (including clinicians, ethicists, data scientists, legal experts, and patient representatives) to review and monitor AI initiatives. Actively address potential biases in algorithms and data to prevent exacerbating health disparities. Strive for transparency in how AI tools work (explainability) and define clear lines of accountability when things go wrong.
Leadership Lens: Patient trust is the absolute bedrock of healthcare. Any technology perceived as opaque, biased, or unsafe will face insurmountable resistance. As leaders, we must champion responsible innovation, ensuring AI is deployed ethically and equitably. This isn't a compliance checkbox; it's fundamental to our license to operate.
4. People & Process Integration: Enabling Human + Machine Collaboration
Even the most brilliant AI tool will fail if the people meant to use it aren't prepared, or if it disrupts rather than enhances established workflows. AI adoption is as much a human change management challenge as a technological one.
The Work: Integrate change management planning into AI projects from the very beginning. Invest significantly in training and upskilling your workforce – clinicians need to understand how to use AI tools effectively and interpret their outputs, while support staff roles may evolve. Critically evaluate and redesign clinical and operational workflows to incorporate AI seamlessly, rather than just layering it on top. Foster open communication and collaboration between technical teams and clinical end-users throughout the development and implementation process. Address fears and anxieties transparently.
Leadership Lens: My experience implementing large systems like Electronic Medical Records highlighted painful lessons about neglecting workflow integration and change management. Clinician buy-in, earned through genuine collaboration and demonstrating value, is make-or-break for adoption. Leaders must champion a culture supportive of learning and adapting to new ways of working.
5. Technology & Implementation: Choosing Wisely, Integrating Effectively
Selecting the right AI tools and ensuring they integrate smoothly with your existing technological ecosystem is critical for operational success.
The Work: Clearly define the technical requirements based on your strategic objectives and workflow needs. Carefully evaluate potential solutions, whether building in-house or buying from vendors (consider factors like validation, interoperability, support, and total cost of ownership). Prioritize solutions that can integrate effectively with your existing EMR and IT infrastructure. Plan for rigorous testing and clinical validation in your specific environment before broad deployment. Implement in phases where possible, allowing for learning and adjustment. Ensure robust plans for ongoing technical support, monitoring, and model updates.
Leadership Lens: Having evaluated technology initiatives from both consulting (McKinsey) and operational (CEO) perspectives, I emphasize due diligence. Avoid being swayed solely by impressive demos. Focus on proven clinical validation, seamless integration capabilities, and long-term vendor viability. The technical choices made here have long-lasting operational and financial implications.
6. Measurement & Iteration: Defining Success and Learning Continuously
How will you know if your AI initiative is successful? Defining success metrics upfront and building in mechanisms for continuous monitoring and improvement is essential.
The Work: Before launching, define clear Key Performance Indicators (KPIs) aligned with your initial strategic goals – these could be clinical (e.g., diagnostic accuracy improvements, reduced complication rates), operational (e.g., reduced wait times, cost savings), and/or related to user experience (e.g., clinician satisfaction). Implement mechanisms to track these KPIs rigorously. Actively solicit and incorporate feedback from end-users. Foster a culture where it's safe to report issues and where data drives ongoing iteration and refinement of the AI tools and processes. Be prepared to adapt or even pivot based on real-world performance.
Leadership Lens: In both scientific research and running large organizations, the principle holds: you can't manage what you don't measure. Defining success clearly and tracking progress relentlessly is fundamental. AI is not a "set it and forget it" technology; it requires ongoing vigilance and a commitment to continuous learning and improvement, driven by data and user feedback.
Leading the Way
Successfully integrating AI into healthcare is not merely a technical challenge; it's a profound strategic undertaking. These six pillars – Vision, Data, Ethics, People, Technology, and Measurement – are interconnected and require dedicated leadership attention. By thoughtfully addressing each one, and particularly by starting with a clear internal vision to guide engagement with the burgeoning AI market, healthcare leaders can move beyond reactive fragmentation. They can build a solid foundation for leveraging AI responsibly and effectively, ultimately advancing our shared goal of delivering better, safer, and more accessible care.
Comments