Beyond the Pilot: A Practical Framework for Building a Scalable AI Roadmap in Your Health System
- Dr Ajay Bakshi
- Apr 28
- 4 min read
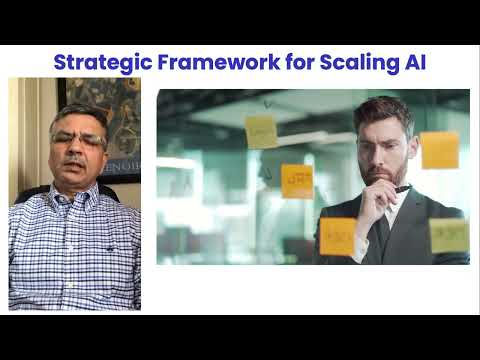
Artificial intelligence promises to revolutionize healthcare, offering tantalizing glimpses of enhanced diagnostics, optimized workflows, and improved patient outcomes. Many health systems have dipped their toes in the water, launching pilot projects that often demonstrate technical success in isolated settings. Yet, a common frustration echoes through boardrooms and hospital corridors: why do so many promising AI initiatives get stuck in the 'pilot trap,' failing to scale and deliver system-wide value?
The reality is that moving AI from contained experiments to enterprise-wide transformation requires more than just effective algorithms. It demands a fundamental shift in thinking – from tactical trials to strategic integration. As highlighted by recent HFS Research, widespread "pilot fatigue" is setting in, often because the initial value demonstrated isn't clearly linked to core strategic KPIs, leading leadership to view AI as a minor efficiency play rather than a true engine for transformation. Scaling AI successfully requires a deliberate, practical, and strategic roadmap.
Based on insights from industry analysis and experience navigating AI adoption in complex healthcare environments, here’s a framework focusing on the core elements needed to build that scalable roadmap:
1. Escaping the 'Pilot Trap': Aligning AI with Strategic Imperatives
The first hurdle is ensuring AI initiatives are not merely interesting technological explorations but are deeply intertwined with the organization's core mission and strategic objectives. Pilots often stall because this link is missing.
● Redefine Value: Move beyond measuring success solely by task efficiency or limited pilot metrics. Anchor every AI initiative to measurable improvements in strategic goals: enhancing specific clinical outcomes, significantly improving operational efficiency metrics (like patient throughput or reduced readmissions), boosting patient experience scores, or creating new revenue streams. When AI’s value is articulated in the language of core business objectives, securing buy-in for scaling becomes much more feasible.
● Strategic Fit Assessment: Before scaling any pilot, rigorously assess its alignment with long-term organizational priorities. Does it address a critical bottleneck? Does it support a key strategic growth area? Does it enhance your competitive positioning? If the answer is unclear, scaling is premature.
2. Building the Foundation: Readiness Beyond the Algorithm
A brilliant algorithm running on inadequate infrastructure or fueled by poor-quality data will inevitably fail at scale. HFS Research and others rightly point out that messy data and disconnected systems are often the silent killers of AI scaling ambitions. A strategic roadmap must prioritize foundational readiness.
● Honest Baseline Audit: Conduct a thorough assessment (as suggested by frameworks like the one proposed by WEF) of your current state:
○ Data Infrastructure: Is your data accessible, interoperable (e.g., leveraging FHIR standards), and governed effectively?
○ Data Quality: Are processes in place to ensure data accuracy, completeness, and representativeness to avoid bias?
○ Technology Stack: Does your existing tech environment support the integration and deployment of AI tools at scale?
○ Talent & Skills: Do your clinical, IT, and operational teams possess the necessary skills to implement, utilize, and manage AI tools effectively?
● Infrastructure Investment: Scaling AI is not just a software project; it often requires significant investment in building a robust digital core – potentially including cloud integration, federated data architectures, and data quality pipelines. This infrastructure isn't just overhead; it's the essential plumbing for any enterprise-wide AI ambition.
3. The Roadmap Itself: Prioritization, Governance, and Engagement
With strategic alignment and foundational readiness addressed, the roadmap can focus on what to scale and how to manage it responsibly.
● Impact-Driven Prioritization: Move beyond simply scaling the easiest pilots. Develop a clear framework to prioritize initiatives based on potential system-wide impact, alignment with strategic goals, technical feasibility (considering foundational readiness), and manageable risk. Frame this not just as an IT decision but a core strategic choice.
● Proactive Governance: Robust governance is not red tape; it's an enabler of trust and responsible scaling. Establish clear policies and oversight mechanisms before widespread deployment. Address crucial questions proactively: Who is accountable for AI outputs? How will bias be monitored and mitigated? How will transparency be maintained, especially with "black box" algorithms? How will patient privacy be ensured? Involving legal, clinical, and IT stakeholders is essential.
● Stakeholder Engagement: Successful scaling requires buy-in across the organization. Engage clinicians, administrators, IT teams, and even patients early and often (as WEF also recommends). Understand their workflows, address their concerns, and co-design integration strategies. AI tools forced onto unwilling or unprepared users rarely achieve their potential.
4. Addressing the Human Factor: Overcoming Organizational Inertia
Often, the most significant barrier isn't technological; it's organizational. Fear of disruption, unclear ownership, siloed decision-making, and general resistance to change can cripple even the best-laid plans.
● Strong Leadership & Vision: C-suite sponsorship is critical. Leaders must champion the AI strategy, clearly communicate the vision, and empower teams to execute.
● Clear Accountability: Define who owns the AI strategy, who is responsible for implementation, and how decisions are made. Ambiguity breeds inaction.
● Invest in Change Management: Treat AI adoption as a significant change initiative. Invest in training, communication, and support to help staff adapt workflows and build confidence in new tools. Address concerns about job roles transparently.
Conclusion: Moving from Experimentation to Transformation
Successfully scaling AI in healthcare demands navigating a complex interplay of clinical realities, technical infrastructure, executive strategy, and organizational change – challenges I've experienced firsthand as a clinician, CEO, and AI innovator. This framework provides a strategic lens, but applying it requires translating these concepts into concrete actions within your specific environment.
If your leadership team is wrestling with these multifaceted challenges and seeking clarity on your path forward, perhaps an initial conversation could prove valuable. You can reach me at drajaybakshi@gmail.com.
Kommentare